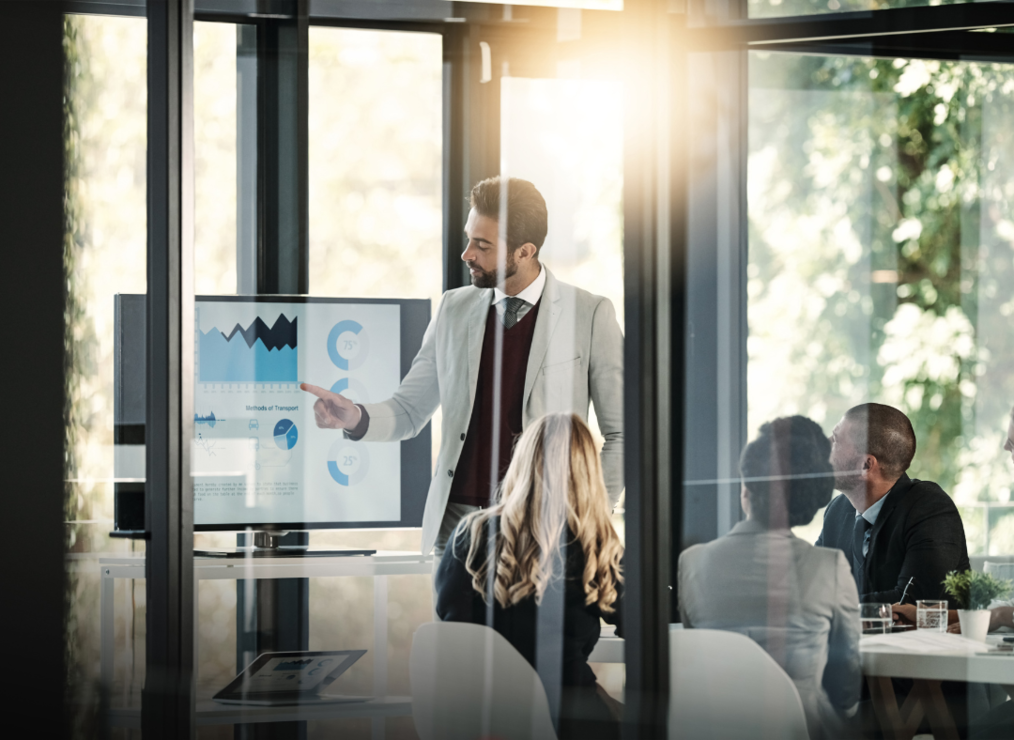
Data-Driven Leadership: Navigating the Changing Landscape of Decision-Making
Jan. 6 2024In the fast-paced world of business, how decisions are made at the executive level is experiencing a noteworthy shift. The integration of Artificial Intelligence (AI) and Large Language Models (LLMs) is steering us away from gut instincts, leading us toward more sophisticated decision-making grounded in data. This article explores the tangible impacts of this change, particularly in terms of how it shapes strategic planning, operational efficiency, and the overall resilience of organizations.
Foundations of Intelligence: Defining Artificial Intelligence and Large Language Models
- Artificial Intelligence involves creating computer systems capable of tasks typically associated with human intelligence, such as learning, problem-solving, and understanding natural language. It encompasses subfields like machine learning and computer vision.
- Large Language Models, a subset of AI, are massive neural network-based models adept at understanding and generating human-like language. Trained on extensive datasets, they play a key role in tasks like natural language understanding and text generation, contributing to advancements in AI applications related to human communication.
The Role of Data Analytics in Executive Decision-Making
-
Informed Strategic Planning
Executives now possess the ability to foresee market shifts through the utilization of real-time data insights. This capability empowers them to make decisions informed by data that surpass conventional market analysis. By synchronizing strategies with up-to-the-minute data, executives can discern emerging trends, market fluctuations, and potential opportunities. This proactive approach to strategic planning enables organizations to swiftly adapt, seize advantages in market dynamics, and navigate unfamiliar territories with heightened confidence.
-
Real-time Optimization
Data analytics enables real-time adjustments to marketing campaigns and operational strategies. This dynamic optimization proves crucial in the ever-changing business landscape where conditions can swiftly evolve. Executives leverage data-driven insights to allocate resources efficiently, promptly respond to emerging trends, and fine-tune operational strategies as required. This agility guarantees optimal performance and responsiveness, ensuring that organizations remain competitive and adaptive amid the continual evolution of market conditions.
-
Proactive Strategies
Gaining insights into customer behaviors and preferences through data analytics equips executives with a substantial competitive advantage. Armed with an understanding of customer trends, executives can proactively mold strategies to meet customer expectations. This not only boosts customer satisfaction but also ensures a competitive edge. Executives can foresee shifts in consumer preferences, initiate targeted campaigns, and customize products and services to align with the evolving needs of their customer base.
-
Risk Mitigation
Predictive analytics stands as a cornerstone in anticipating potential risks and implementing measures to safeguard the interests of the organization. Through the analysis of historical data and the identification of patterns, executives can proactively assess and mitigate risks. Whether dealing with financial risks, supply chain disruptions, or other uncertainties, predictive analytics enables executives to make decisions backed by data, enhancing risk management strategies. This proactive approach minimizes the impact of potential threats and contributes significantly to the overall resilience of the organization.
Implementing a Data-Driven Culture in the C-Suite
-
Leadership Alignment
Executives must actively engage with data analytics principles to set a precedent for the organization's transition to a data-driven culture. This involves not only acknowledging the value of data but also integrating data-driven decision-making into the leadership mindset. Executives should champion data initiatives, encourage experimentation, and lead by example in leveraging data to inform decisions. Leadership alignment is crucial for fostering a culture where data is viewed as a strategic asset, and its insights are integral to decision-making processes at all levels.
-
Data Literacy
Ongoing education and training in data analytics are crucial for executives to interpret and utilize data effectively. Building data literacy within the C-suite ensures that executives can understand, interpret, and critically evaluate data-driven insights. This involves providing training programs, workshops, and resources to enhance the data literacy skills of executives. A data-literate leadership team is better equipped to ask the right questions, make informed decisions, and communicate effectively about data-driven strategies.
-
Security Challenges
Navigating data security complexities is essential to ensure compliance with regulations and maintain customer trust. Executives need to be actively involved in shaping robust data security policies and practices. This includes understanding the regulatory landscape, implementing encryption measures, and fostering a culture of data privacy within the organization. Addressing security challenges is critical for maintaining the integrity of data-driven decision-making processes and ensuring that data is handled responsibly and ethically.
-
Data Team Dynamics
Data teams, adopting software development practices, are central to creating data products that drive business strategy and operational efficiency. Executives should foster collaboration between data teams and other departments, breaking down silos and ensuring cross-functional cooperation. By adopting agile development practices, data teams can iterate quickly, respond to changing business needs, and deliver valuable data products that inform strategic decision-making. Executives play a key role in promoting a collaborative environment where data teams are integrated into the broader organizational strategy.
-
Efficiency with Less Data
Prioritizing small data and workload offloading not only optimizes resources but also aligns with overarching goals of cost management and operational agility. Executives are encouraged to champion a focus on extracting meaningful insights from smaller datasets, fostering efficiency in data collection and processing. This approach ensures organizations are not overwhelmed by data volume but instead prioritize the quality and relevance of the data they collect. By embracing efficient data practices, executives actively contribute to cost-effectiveness and enhance the organization's ability to swiftly adapt to changing market conditions.
Benefits of Data-Driven Decision Making
-
Accuracy and Reliability
The foundation of reliability and precision rests upon the integrity of data. Guaranteeing the dependability of the data used ensures that decisions are firmly grounded in verifiable facts, ultimately leading to outcomes that are not only more precise but also inherently trustworthy.
-
Objective Decision Making
Fostering objective decision-making necessitates the elimination of biases and subjectivity from the decision-making process. Creating an environment where decisions are impartial and free from personal inclinations cultivates a culture where objectivity reigns supreme, allowing for choices grounded solely in factual data.
-
Improved Efficiency
The reliance on data as a guiding force in decision-making acts as a catalyst for heightened efficiency. The shift from speculative decision-making to a data-centric approach drastically reduces guesswork, culminating in a more streamlined and efficient decision-making process. The result is a faster and more responsive organizational apparatus.
-
Competitive Advantage
The strategic deployment of data bestows organizations with a potent weapon – a competitive edge. By effectively harnessing data, organizations gain insightful understanding of market dynamics, customer behaviors, and emerging trends. This not only positions them ahead of the competition but also empowers informed decision-making, creating a distinct advantage in a competitive landscape.
Common Challenges with Data-Driven Decision Making
Achieving successful data-driven decision-making is not without its hurdles. Organizations navigating the landscape of data analytics encounter several challenges that demand strategic solutions. Addressing these challenges is pivotal for ensuring the reliability and efficacy of the decision-making process.
-
Data Limitations
- Issue: Many decisions require a holistic view that extends beyond a single dataset. Relying solely on existing datasets may lead to an incomplete or inaccurate understanding of the situation.
- Solution: Organizations should explore data marketplaces and leverage cloud platforms to enrich existing data. Embracing diverse data sources helps create a more comprehensive and accurate picture, enabling more informed decision-making.
-
Biased Data
- Issue: Without proper context and analysis, decisions may be based on biased data, leading to incorrect assumptions and potentially flawed outcomes.
- Solution: Organizations must consider additional factors, such as customer feedback, market trends, and competitive analysis, to augment and enrich existing data. Implementing a diverse approach to data collection helps counteract biases and promotes more objective decision-making.
-
Data Privacy Concerns
- Issue: With the increasing availability of customer information, data privacy becomes a major concern. Organizations need to balance the benefits of data-driven decision-making with the need to protect sensitive customer information.
- Solution: Implementing robust measures for data protection and compliance with evolving regulations is crucial. Tools that provide granular control over data access and usage, especially in self-service solutions, contribute to maintaining data privacy.
-
Data Quality
- Issue: Poorly managed data can lead to inaccurate results and misleading conclusions, hindering the decision-making process and potentially resulting in erroneous outcomes.
- Solution: To ensure data quality, organizations should track metrics that assess the accuracy, timeliness, and completeness of their data. Regular data audits and cleaning processes contribute to maintaining high-quality data, providing a reliable foundation for decision-making.
Organizations tackling challenges in data-driven decision-making must embrace a comprehensive strategy. This involves integrating technology, streamlining processes, and fostering a cultural commitment to data excellence. Proactively addressing these issues enhances organizational capabilities, ensuring reliable and unbiased insights aligned with goals. This proactive stance cultivates a data-centric culture, instilling trust among decision-makers and facilitating more confident and effective decision-making outcomes.
5 Key Steps to Making Better Data-Driven Decisions
A thorough and encompassing approach for embedding data-driven decision-making seamlessly into organizational practices:
-
Define the Problem
- Importance: Every data project is fundamentally a business project. Before making decisions, it is crucial to identify and thoroughly understand the problem at hand. This involves defining goals and objectives, understanding the context of the situation, and ideating potential solutions.
- Collaboration: To achieve this, businesses must foster collaboration between data teams and business users. This ensures that the business problem is front and center from the outset, and solutions proposed from data teams align with improving outcomes that business users aim to influence.
-
Collect Relevant Data
- Importance: Once the true business use case is identified, the next step is to gather relevant data that can enhance the decision-making process related to the challenge at hand.
- Diverse Data Sources: Gathering data from both internal and external sources, such as customer surveys, industry reports, market research, and historical trends, is essential. Leveraging the ease of bringing data together in a cloud data platform facilitates this process.
-
Analyze the Data
- Importance: After capturing, cleaning, and organizing relevant data, the next step is the actual analysis to unearth patterns, outliers, anomalies, and trends that can inform decision-making.
- Empower Business Users: This phase should include empowering not only data professionals but also business users to engage directly with the data. Early engagement helps identify potential data gaps, new use cases, and training opportunities, driving adoption and value. Tools like ThoughtSpot make it easy for business users to engage with data without requiring technical skills.
-
Develop and Implement a Plan
- Importance: Once the analysis is complete, it's time to develop a plan to address the identified problem. This involves creating strategies, setting achievable goals and objectives, defining the right data Key Performance Indicators (KPIs), and establishing a timeline for completion.
- Involving Business Stakeholders: It is crucial to ensure that the plan leads to the desired outcomes. Involving business stakeholders from the outset and continuing their engagement during the implementation phase is vital for driving real adoption and meaningful impact.
-
Evaluate the Results
- Importance: The final step in the process is to evaluate the results of the efforts made toward more data-driven decision-making.
- Key Performance Indicators: By tracking key performance indicators, businesses can gauge how their efforts have addressed the specific business challenge and impacted the organization. This evaluation not only improves existing use cases but also provides insight into areas of operations that could be enhanced, contributing to more informed decisions in the future.
Examples of Data-Driven Decisions
-
Amazon's Recommendation System
Amazon's state-of-the-art recommendation system stands as a prime example of the substantial influence exerted by data-driven decision-making. Utilizing advanced AI algorithms, Amazon meticulously scrutinizes customer behavior, purchase history, and preferences. The outcome is a flawlessly tailored product recommendation experience, elevating user satisfaction and substantially augmenting sales. This serves as a compelling illustration of the efficacy of harnessing data insights to inform decision-making processes.
-
Netflix Content Recommendations
At the forefront of personalized entertainment, Netflix takes the lead by leveraging machine learning algorithms to analyze viewer preferences and viewing patterns. With an extensive reservoir of data at its disposal, Netflix provides remarkably accurate content recommendations. This data-driven strategy not only enhances content curation but also heightens customer satisfaction through a tailored viewing experience aligned with individual preferences. Netflix's triumph underscores the effectiveness of data-driven decision-making in the domain of content delivery.
-
Google's AdWords and Predictive Analytics
Google's AdWords serves as an exemplary model of data-driven decision-making within the sphere of online advertising. By integrating predictive analytics, AdWords dynamically refines ad placements, leveraging user behavior and historical data. This real-time optimization strategy underscores the fundamental role played by data analytics in shaping robust advertising strategies. Google's approach accentuates the significance of maintaining a leading position in the digital advertising landscape, emphasizing continuous adaptation driven by invaluable data insights.
-
Tesla's Autonomous Driving Technology
Tesla's pioneering advancements in autonomous driving are propelled by the ongoing analysis of data extracted from its extensive fleet of vehicles. Utilizing this vast repository of information, Tesla employs a combination of AI and data analytics to iteratively enhance and update autonomous driving features. This serves as a prime example of the influential role of data-driven decision-making in fostering innovation within the highly competitive automotive industry. Tesla's steadfast commitment to harnessing data ensures that its autonomous technology consistently leads the industry, setting high standards for technological excellence.
-
Facebook's Ad Targeting
Facebook's proficiency in targeted advertising derives from its skillful utilization of user data. Through meticulous data analytics, Facebook tailors ad targeting strategies to align with user behaviors and preferences. This proactive approach to marketing stands as a prime example of the profound impact of data-driven decision-making in comprehending and engaging with customers. Facebook's success in the realm of ad targeting underscores the strategic advantage enjoyed by businesses that harness data insights, enabling the formulation of personalized and highly effective marketing strategies.
Conclusion
The combination of AI, LLMs, and data analytics stands out as a transformative force. Executives are increasingly turning to data to guide their decisions, ushering in an era of more informed and nuanced leadership. By instilling a data-driven mindset among leaders and proactively addressing challenges, organizations position themselves for decision-making marked by precision, objectivity, and a distinct competitive advantage. In an era where critical thinking and strategic data utilization define success, organizations adept at navigating this change are not just adapting but thriving.